The Evolution of AI: From Expert Systems to Neuromorphic Engineering
In the ever-evolving landscape of technology, the term “Artificial Intelligence (AI)” has become a mainstay, often used to describe a future-forward approach to problem-solving and innovation. But what is AI, and how has it evolved to hold such a pivotal role in modern society? In this article, we embark on a journey through the transformative phases of AI, from its nascent stages rooted in expert systems to the promising horizon adorned with neuromorphic engineering.
Definition of Artificial Intelligence (AI)
To truly grasp the AI meaning, we must first understand it as more than just a technological term. Artificial Intelligence AI, also known as machine intelligence, is a branch of computer science that focuses on creating technology capable of autonomous decision-making and actions, essentially simulating aspects of human intelligence. It encompasses a wide array of software and hardware components, including machine learning, expert systems, and certain robotics forms, all working harmoniously to support the AI define narrative.
The Importance of Understanding “What is AI” in Today’s Tech Landscape
Understanding what is AI technology is not just about comprehending a scientific term; it is about appreciating a revolutionary force that has reshaped industries and daily life paradigms. It is the force behind smart homes, predictive analytics, and even the voice assistant responding to your commands. As we delve deeper, we will uncover the layers that make AI the powerhouse it is today.
The Birth of AI: Expert Systems
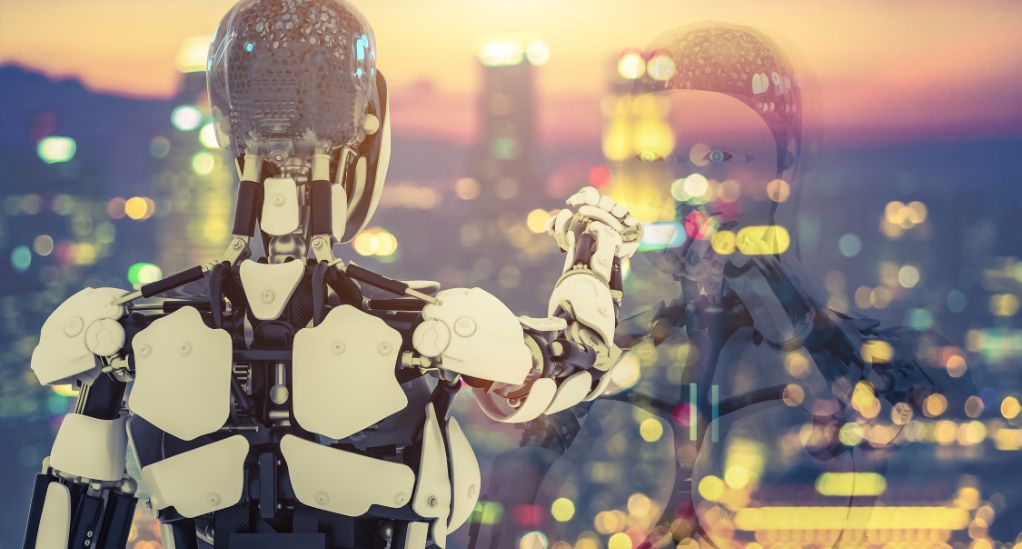
As we trace back the roots of AI, we find ourselves in the 1970s and 1980s, a period marked by the emergence of expert systems. These were the initial attempts to capture and utilize the knowledge of human experts in specific domains.
What is AI Technology in the 1970s and 1980s?
During this period, the focus was on creating systems that could hold a vast amount of information and provide recommendations or make decisions based on that data. These expert systems were designed to simulate the decision-making process of human experts, offering a glimpse into the potential of what AI technology could achieve.
Key Characteristics of Expert Systems in the 1970s and 1980s
Feature | Description |
---|---|
Knowledge Base | A database storing all the information, rules, and data the system uses to make decisions. |
Inference Engine | The component that applies the rules to the known data to deduce new information. |
User Interface | The platform through which users interact with the expert system, often through a series of questions and answers. |
The Role and Significance of Expert Systems in the Early Stages
Expert systems played a crucial role in setting the stage for more advanced AI technologies. They were used in various fields including, but not limited to, healthcare for diagnostic purposes, finance for investment analysis, and engineering for complex problem-solving.
Expert Systems Applications Across Different Industries
Industry | Application of Expert Systems | Benefits |
---|---|---|
Healthcare | Diagnostic assistance | Improved accuracy and efficiency in diagnosing diseases |
Finance | Investment analysis | Enhanced decision-making in investments through data analysis |
Engineering | Problem-solving | Facilitating solutions to complex engineering problems through data analysis |
The Growth of AI: Machine Learning and Deep Learning
As we transition from the foundational era of expert systems, we venture into the transformative phases of machine learning and deep learning, which have significantly shaped the AI meaning in contemporary times.
AI Meaning in the Context of Machine Learning
Machine learning (ML), a subset of AI, introduced a paradigm shift in artificial intelligence AI. Unlike expert systems that relied on predefined rules and databases, ML focuses on building models that allow computers to learn from data. This learning process enabled computers to make predictions or decisions without being explicitly programmed to perform a task, thus broadening the AI define spectrum.
Distinction Between Expert Systems and Machine Learning
Aspect | Expert Systems | Machine Learning |
---|---|---|
Basis | Predefined rules and databases | Data-driven learning |
Flexibility | Limited to the knowledge base | Adaptable through learning from new data |
Application | Specific domains | Wide-ranging applications across various fields |
The Rise of Deep Learning and Its Current Status
Deep learning took machine learning a step further by stacking machine learning algorithms in a hierarchy of increasing complexity and abstraction. This iterative approach has become the most sophisticated AI architecture in use today, capable of recognizing patterns in data with a deep level of nuance and precision.
Subsection: Deep Learning Architectures
- Convolutional Neural Networks (CNNs): Primarily used in image and video recognition.
- Recurrent Neural Networks (RNNs): Suitable for sequence prediction tasks such as speech recognition.
- Generative Adversarial Networks (GANs): Employed in generating new data that is similar to the training data.
How Deep Learning Changed the AI Define Narrative
Deep learning has not just been a technological advancement; it has been a narrative changer. It brought forth applications that were once considered science fiction, such as deepfake videos and sophisticated voice synthesis technologies. It has expanded the boundaries of what is AI, offering a glimpse into a future where AI technologies can create, innovate, and even imagine, akin to human intelligence.
The Advent of Neuromorphic Engineering
As we forge ahead in our exploration of the AI landscape, we arrive at a frontier that promises to redefine the boundaries of what is AI technology: neuromorphic engineering. This emerging field seeks to mimic the human brain’s architecture, paving the way for an AI future that is more intuitive, adaptive, and efficient.
What is Neuromorphic Engineering?
Neuromorphic engineering stands at the intersection of neuroscience and artificial intelligence AI. It is an interdisciplinary field that focuses on designing specialized hardware and algorithms optimized for low power consumption and real-time processing, aiming to emulate the human brain’s architecture. This approach promises to usher in a new era where AI systems are not just tools but entities capable of learning and evolving autonomously.
The Role of Neuromorphic Engineering in Emulating the Human Brain’s Architecture
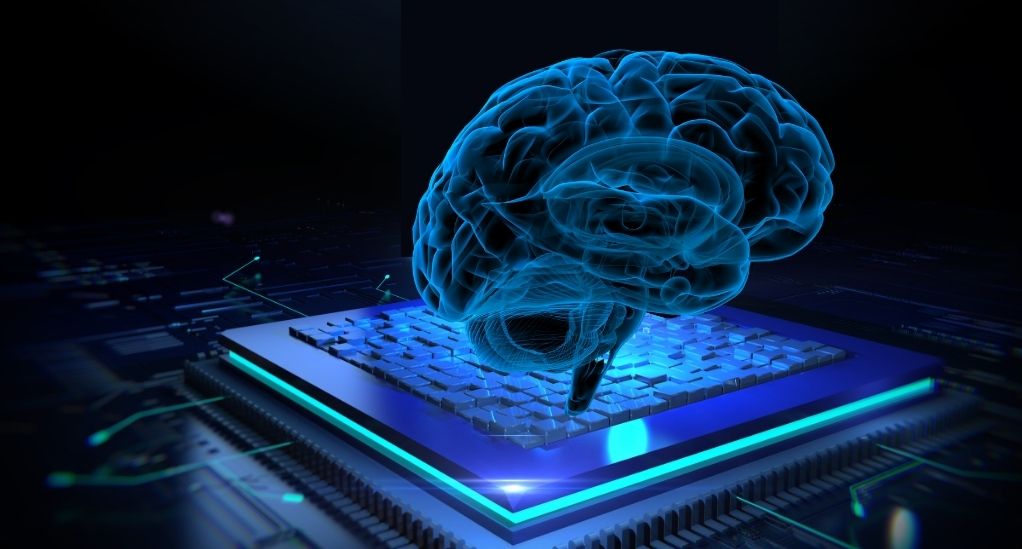
Neuromorphic engineering seeks to bring AI closer to human cognitive processes by leveraging the brain’s architecture. This involves creating systems that can process information with high speed, adaptive learning capabilities, and energy-efficient manners, thus redefining the AI meaning in the context of hardware advancements.
Subsection: The Potential of Neuromorphic Computing
- Energy Efficiency: Neuromorphic systems are designed to consume less power compared to traditional AI systems, making them more sustainable.
- Real-time Processing: These systems can process information in real-time, enhancing responsiveness and efficiency.
- Adaptive Learning: Neuromorphic systems can learn and adapt, evolving to become more intelligent over time.
The Potential of Neuromorphic Engineering in Revolutionizing AI Applications
As we stand on the cusp of a neuromorphic revolution, it is essential to explore the potential applications of this technology. From healthcare to autonomous vehicles, the possibilities are endless, promising a future where AI is more integrated and harmonious with human existence.
AI in Business Applications
As we navigate further into the intricate world of artificial intelligence AI, it becomes imperative to delve into its applications in the business realm. AI has not just remained a theoretical concept; it has permeated various business sectors, revolutionizing operations and offering unprecedented efficiencies.
Overview of AI Use Cases in Business
AI has found a home in numerous business applications, ranging from customer service to supply chain management. It has facilitated smarter decision-making, predictive analytics, and automation, fundamentally altering how businesses operate and compete in a dynamic market landscape.
Deep Dive into Technologies such as Artificial Neural Networks, Speech Recognition, and Natural Language Generation
To truly grasp the AI meaning in business, we need to understand the technologies that power these applications. Let’s delve deeper into a few pivotal technologies and their roles in reshaping the business landscape.
Subsection: Artificial Neural Networks in Business
- Predictive Analytics: Leveraging neural networks to analyze data and predict future trends, helping businesses to make informed decisions.
- Fraud Detection: Utilizing neural networks to identify patterns and anomalies in data, aiding in the early detection of fraudulent activities.
Subsection: Speech Recognition and Natural Language Generation in Business Communication
- Voice-Activated Assistants: Enhancing customer service through voice-activated assistants that can understand and respond to natural language queries.
- Chatbots: Facilitating seamless customer interactions through chatbots capable of understanding and generating natural language responses.
Real-World Examples of AI Transforming Business Operations
As we explore the real-world implications of AI in business, we find numerous case studies that showcase AI’s transformative power. From AI-powered recommendation systems in e-commerce platforms to predictive maintenance in manufacturing, AI has been a game-changer.
The Different Faces of AI: Weak AI vs. Strong AI
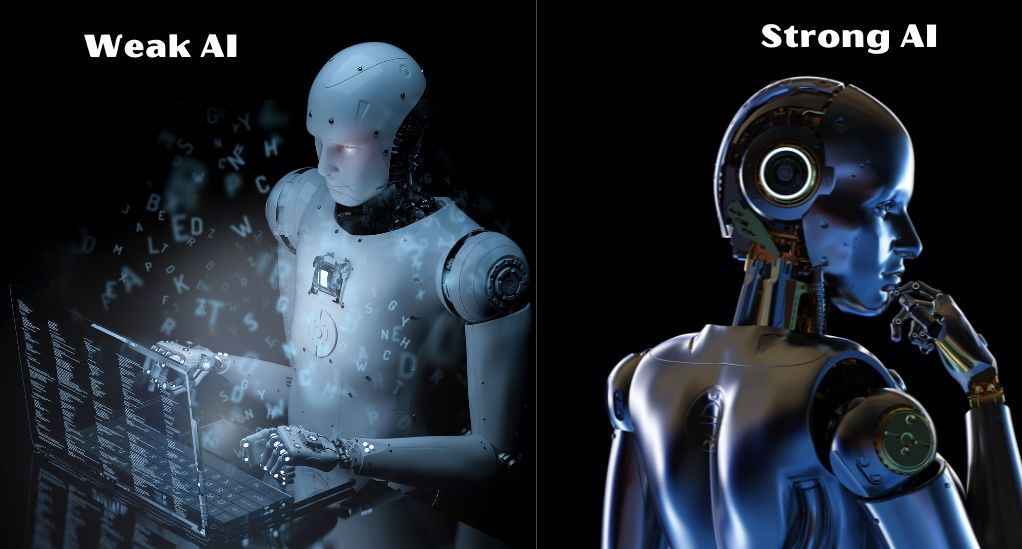
As we continue our exploration of the artificial intelligence AI landscape, it is essential to understand the different classifications that have emerged in the discourse over the years. These classifications not only help in understanding what is AI technology at different levels of sophistication but also aid in envisioning the potential trajectories AI could take in the future.
Understanding the Classifications: Weak AI, Strong AI, and Super AI
The AI landscape is often delineated into three primary categories: weak AI, also known as narrow AI; strong AI, synonymous with general AI; and a futuristic concept, super AI. Each category represents a different level of AI’s cognitive abilities, offering a structured way to understand the AI meaning at various developmental stages.
The Capabilities and Limitations of Each Classification
Each classification of AI comes with its own set of capabilities and limitations, defining the boundaries of what AI can achieve at different stages of its evolution. Let’s delve deeper into each classification to understand their potential and the challenges they pose.
Subsection: Weak (Narrow) AI
- Capabilities: Expertise in specific tasks, efficiency, and accuracy in data processing.
- Limitations: Lack of understanding and learning abilities beyond the predefined tasks.
Subsection: Strong (General) AI
- Capabilities: Autonomous learning, understanding wide set of stimuli, potential to revolutionize technology.
- Limitations: Currently theoretical, poses ethical and safety concerns.
Subsection: Super AI
- Capabilities: Surpassing human intelligence, potential to solve complex global issues.
- Limitations: Hypothetical, potential existential risk to humanity.
Future Predictions: The Concept of Super AI
As we venture into the realm of future predictions, the concept of super AI stands as a beacon of both immense potential and caution. It represents a future where AI could not only revolutionize technology but also redefine the very fabric of human existence, bringing forth ethical, philosophical, and safety concerns that are paramount in the AI define discourse.
The Current Reality of AI
As we further our journey into the world of artificial intelligence AI, it becomes essential to ground ourselves in the current reality of AI. While the future holds immense potential, understanding what is AI technology capable of today helps in distinguishing between science fiction and the tangible advancements that are shaping our world.
Debunking the Myths: AI in Science Fiction vs. Real-World AI Systems
In the realm of science fiction, AI often takes the form of sentient beings with consciousness and emotions. However, the current reality is far from this depiction. Today’s AI systems are incredibly advanced but still operate based on algorithms and patterns, lacking consciousness and the ability to feel emotions.
The Limitations of Current AI Systems: Lack of Consciousness, Emotions, and Self-Awareness
Despite the remarkable advancements, current AI systems have their limitations. They lack consciousness, the ability to understand emotions truly, and self-awareness. These limitations delineate the boundary between AI and human intelligence, defining the AI meaning in its current state.
Subsection: The Challenges in Achieving True AI Consciousness
- Understanding Emotions: Current AI systems can mimic understanding emotions through sentiment analysis but lack true emotional understanding.
- Self-Awareness: AI lacks self-awareness, operating based on programmed algorithms without a true understanding of self.
- Ethical Considerations: The journey towards achieving true AI consciousness brings forth a plethora of ethical considerations, including the rights of AI and the potential impact on society.
The Role of AI in Data Analysis and Language Translation
Despite the limitations, AI has carved out a significant role in various fields, including data analysis and language translation. Through machine learning and deep learning algorithms, AI systems can analyze vast datasets, offering insights and facilitating decision-making processes.
The Future of AI: A Glimpse into What Lies Ahead
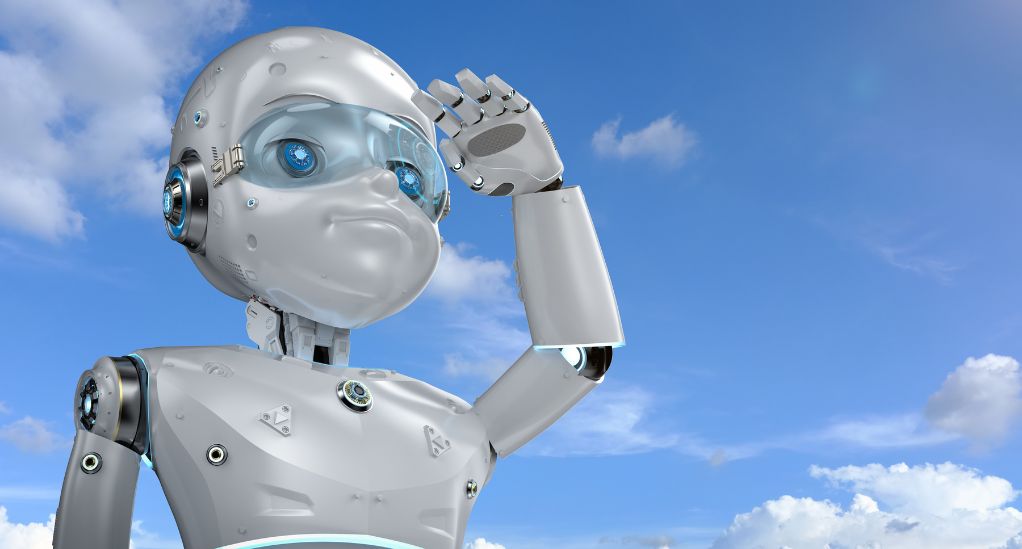
As we reach the final leg of our journey through the landscape of artificial intelligence AI, we stand on the threshold of the future, a realm brimming with possibilities and opportunities. In this section, we will venture into the speculative territory of what lies ahead in the AI journey, drawing from the rich history of its evolution to envision the potential developments that could redefine what is AI technology in the coming years.
The Evolving Stages of Artificial Intelligence
The journey of AI has been marked by continuous evolution, with each stage bringing forth new advancements and capabilities. As we look ahead, it is anticipated that AI will continue to evolve, potentially leading to breakthroughs that could once again redefine the AI meaning, bringing it closer to achieving true consciousness and understanding.
Potential Future Developments in AI
Development | Description |
---|---|
Quantum Computing | Leveraging the principles of quantum mechanics to enhance computing power, potentially revolutionizing AI algorithms. |
Conscious AI | The development of AI systems with a level of consciousness, capable of understanding and interpreting the world in a manner akin to humans. |
AI and Space Exploration | Utilizing AI technologies in space exploration, potentially aiding in the discovery of new celestial bodies and phenomena. |
The Demand for Faster, More Energy-Efficient Information Processing
As AI systems become more complex, there is an increasing demand for faster and more energy-efficient information processing solutions. Neuromorphic engineering and quantum computing stand as promising avenues to meet this demand, potentially revolutionizing the AI landscape with unprecedented computational capabilities.
Subsection: The Role of Neuromorphic Engineering in Future AI Developments
- Energy Efficiency: Neuromorphic systems promise to deliver high computational power with lower energy consumption, paving the way for more sustainable AI technologies.
- Real-Time Processing: The ability to process information in real-time stands as a pivotal feature in the next generation of AI systems, offering responsiveness and efficiency in various applications.
Predictions for the Future: What Can We Expect from AI in the Coming Years?
As we venture into the speculative realm of future predictions, we find a landscape rich with possibilities. From AI systems capable of creating art to the potential development of conscious AI beings, the future holds a plethora of opportunities that could redefine the AI define narrative.
Conclusion
As we navigated the complex landscape of artificial intelligence (AI), we have unearthed its rich history, current realities, and the boundless potential it harbors for the future. While AI stands as a beacon of human ingenuity, it also brings forth critical ethical and societal dilemmas that necessitate careful consideration and responsible stewardship. As we stand at this juncture, it is imperative to foster a future where AI harmonizes with the betterment of society, guided by ethical principles and a deep understanding of its transformative power. Let us embrace the AI journey with informed perspective and hopeful anticipation, steering towards a future that is both innovative and inclusive.
Leave a Reply