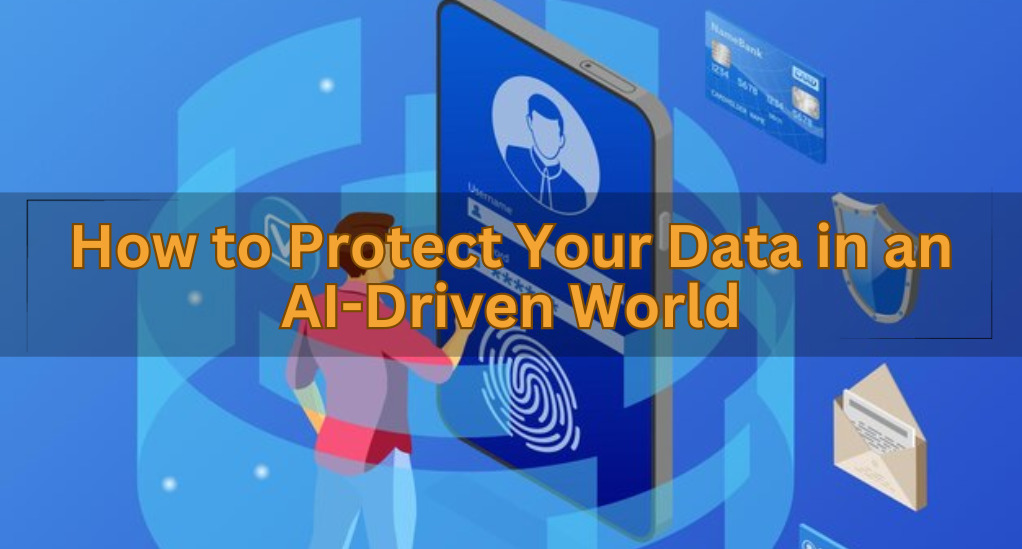
How to Protect Your Data in an AI-Driven World
In today’s rapidly evolving technological landscape, Artificial Intelligence (AI) stands out as one of the most transformative forces. From smart homes and personalized healthcare to advanced manufacturing and beyond, AI is reshaping the way we live, work, and communicate. However, with this technological advancement comes a pressing concern: the safety and privacy of our personal data.
The Rise of AI and Its Implications on Data Privacy
AI systems, by their very nature, thrive on data. They require vast amounts of information to learn, adapt, and make decisions. Every time we use a voice assistant, search the web, or even shop online, we feed these systems with more data about our preferences, habits, and behaviors. While this data-driven approach has led to more personalized and efficient services, it also poses significant risks to our privacy.
Consider this: AI algorithms can predict our future actions, gauge our emotional states, and even deduce our health conditions—all by analyzing the digital footprints we leave behind. Such profound insights into our lives, if mishandled or misused, could lead to breaches of privacy, identity theft, or even manipulation.
The Importance of Understanding AI’s Impact on Personal Data
Understanding the relationship between AI and data privacy is not just about recognizing the risks. It’s about empowering individuals with the knowledge and tools to protect their data. As AI systems become more integrated into our daily lives, the line between convenience and intrusion can blur. Hence, it’s crucial for everyone—consumers, businesses, and policymakers—to be informed and vigilant.
The Information Big Bang
As we navigate the digital age, we’re witnessing an unprecedented explosion of data, often referred to as the “Information Big Bang.” This phenomenon is reshaping the digital universe, with the volume of data doubling approximately every two years.
How Data is Doubling Every Two Years
Every click, swipe, and interaction online contributes to this vast reservoir of information. From social media posts and online purchases to IoT devices and wearable tech, every digital action generates data. Moreover, with the proliferation of connected devices, the Internet of Things (IoT) alone is expected to consist of 75 billion devices by 2025, each contributing its share to the data deluge.
Consider the following:
- Social Media: Every minute, users upload 500 hours of video on YouTube, send 41.6 million WhatsApp messages, and post 347,222 stories on Instagram.
- E-commerce: Online shopping transactions, product searches, and customer reviews add to the data pool every second.
- Healthcare: Wearable devices monitor heart rates, sleep patterns, and physical activity, continuously sending this data to the cloud.
Data Generation Every Minute | Volume |
---|---|
YouTube Video Uploads | 500 hours |
WhatsApp Messages | 41.6 million |
Instagram Stories | 347,222 stories |
The Significance of Moore’s Law and Metcalfe’s Law in the AI Era
Two fundamental principles have been driving the growth of technology and, by extension, the explosion of data: Moore’s Law and Metcalfe’s Law.
- Moore’s Law: Proposed by Gordon Moore in 1965, this law posits that the number of transistors on a microchip doubles approximately every two years, leading to an exponential increase in computing power and a decrease in cost per transistor. This has enabled devices to process more data faster and more efficiently than ever before.
- Metcalfe’s Law: This law states that the value of a network is proportional to the square of the number of its users. In simpler terms, as more devices connect to the internet and interact with each other, the value (and the data they produce) grows exponentially.
Together, these laws underscore the rapid advancements in technology and the interconnectedness of devices, leading to the current data explosion. As AI systems leverage this data for learning and decision-making, understanding the origins and growth patterns of this data becomes crucial for anyone concerned about privacy.
The Three “Vs” of Big Data: Volume, Variety, and Velocity
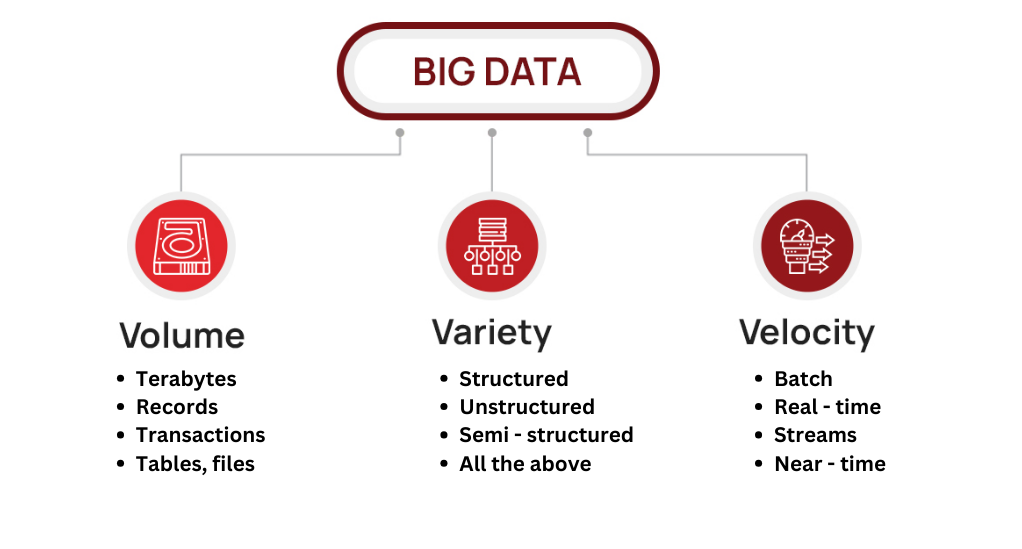
The term “Big Data” has become ubiquitous in today’s digital discourse. But what exactly does it entail? At its core, Big Data is characterized by the three “Vs”: Volume, Variety, and Velocity. These dimensions not only define the nature of the data we deal with but also highlight the challenges and opportunities it presents, especially in the context of AI.
Volume: The Sheer Scale of Data
The first and most apparent characteristic of Big Data is its sheer volume. As previously mentioned, the digital universe is expanding at an unprecedented rate. By 2025, it’s estimated that 463 exabytes of data will be created each day globally. To put that into perspective, one exabyte is equivalent to one billion gigabytes.
This massive volume of data offers a goldmine for AI systems. The more data an AI model has, the better it can learn, adapt, and make accurate predictions. However, managing and storing such vast amounts of data also pose significant challenges, from infrastructure costs to potential security vulnerabilities.
Variety: Diverse Types of Data
Data doesn’t come in a one-size-fits-all format. From structured data like databases and spreadsheets to unstructured data like videos, images, and social media posts, the variety is immense. Each data type provides a unique perspective and can offer different insights when analyzed.
For AI, this variety is both a boon and a challenge. While diverse data types can enhance an AI model’s understanding and accuracy, they also require sophisticated algorithms capable of processing and integrating multiple data formats.
Velocity: The Speed of Data Generation and Processing
In today’s fast-paced digital world, data isn’t just vast and varied; it’s also generated and processed at breakneck speeds. Real-time analytics, instant feedback loops, and live data streams are becoming the norm. For instance, stock trading algorithms analyze market data in microseconds to make buying or selling decisions.
AI systems, especially those in critical sectors like healthcare or finance, need to process data in real-time to deliver timely results. The velocity of data, therefore, underscores the need for AI models that can operate and adapt in real-time, ensuring timely insights and actions.
AI’s Impact on Privacy
The integration of AI into our daily lives has brought about unparalleled conveniences, from personalized content recommendations to advanced health diagnostics. However, this deep entrenchment of AI also raises pressing concerns about individual privacy. As AI systems become more adept at processing and analyzing data, the line between beneficial personalization and invasive surveillance becomes increasingly thin.
How AI-Driven Systems Like Facial Recognition Are Affecting Privacy
One of the most debated AI technologies in the context of privacy is facial recognition. While it offers numerous benefits, such as enhanced security measures and streamlined authentication processes, it also poses significant privacy risks.
Imagine walking into a store, and instantly, the store’s AI system recognizes you, accesses your shopping history, and starts recommending products or even adjusts pricing based on your purchase habits. While this might enhance the shopping experience for some, it’s a stark invasion of privacy for others. In public spaces, unchecked use of facial recognition can lead to constant surveillance, potentially stifling freedom of movement and expression.
The Global Debate on the Ethical Use of AI Technologies
The rapid advancements in AI have outpaced the development of ethical guidelines and regulations. This lag has sparked a global debate on how to ensure the ethical use of AI technologies. Questions about consent, data ownership, and the right to privacy are at the forefront of this discussion.
For instance, should companies be allowed to use AI to analyze employee behaviors without explicit consent? Or, to what extent should governments employ AI surveillance in public spaces? Balancing the benefits of AI with ethical considerations is a challenge that societies worldwide are grappling with.
The Shift from Reactive to Proactive Data Protection
Traditionally, data protection has been reactive, with measures implemented after breaches or misuse. However, in an AI-driven world, this approach is no longer sufficient. There’s a growing emphasis on proactive data protection, where potential threats are anticipated, and protective measures are put in place in advance.
This shift is evident in the rise of privacy-by-design frameworks, where privacy considerations are integrated into the very design and architecture of AI systems. Such proactive measures ensure that privacy is not an afterthought but a foundational principle.
Challenges in AI and Privacy
As we delve deeper into the intersection of AI and privacy, it becomes clear that this convergence presents a myriad of challenges. From technical limitations to ethical dilemmas, understanding these challenges is the first step towards crafting effective solutions.
The Limitations and Failures of AI Systems
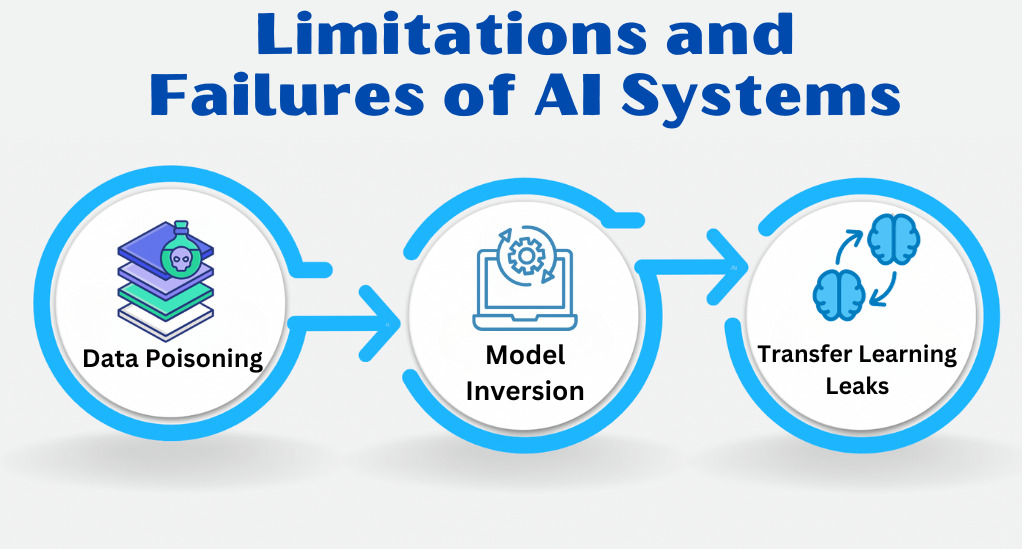
No AI system is infallible. Even the most advanced models can make errors, misinterpret data, or be vulnerable to external manipulations. For instance:
- Data Poisoning: Malicious actors can introduce skewed data into AI training sets, causing the system to make incorrect predictions or decisions. Such vulnerabilities can be exploited to breach privacy or even cause harm.
- Model Inversion: Sophisticated attackers can use the outputs of an AI system to reconstruct the data it was trained on, potentially revealing sensitive information.
- Transfer Learning Leaks: When AI models are trained on one dataset and fine-tuned on another, there’s a risk that information from the original dataset can be inferred, leading to potential privacy breaches.
The Distinction Between General AI Data Issues and Personal Information-Specific Issues
While many challenges in AI revolve around data accuracy, efficiency, and security, privacy concerns are particularly acute when dealing with personal information. Personal data, such as medical records, financial details, or even behavioral patterns, can have profound implications if mishandled or misused.
For instance, while a misclassification of an image in a general dataset might be inconsequential, an incorrect diagnosis based on medical imaging AI could have life-altering consequences. Similarly, the unauthorized access or misuse of personal financial data can lead to identity theft or fraud.
The Evolving Nature of Personal Data
In the traditional sense, personal data referred to identifiable information like names, addresses, or social security numbers. However, in the AI era, even seemingly innocuous data points, like browsing habits or location patterns, can be pieced together to create detailed personal profiles. This expanded definition of personal data complicates privacy protection measures, as even non-traditional data types need to be safeguarded.
Algorithmic Discrimination and Privacy
One of the most critical aspects of privacy in the age of AI is the issue of algorithmic discrimination. AI systems, when trained on biased or unrepresentative datasets, can perpetuate and even exacerbate existing biases and discrimination. This poses a significant threat to privacy, fairness, and social justice.
Understanding the Scope and Implications of Algorithmic Bias
Algorithmic bias occurs when an AI system consistently makes predictions or decisions that favor or disfavor certain groups based on race, gender, age, or other protected characteristics. This can manifest in various domains, such as:
- Criminal Justice: Predictive policing algorithms may unfairly target specific communities, leading to unjust arrests and sentencing disparities.
- Hiring and Employment: AI-powered hiring tools may inadvertently discriminate against candidates from underrepresented groups, leading to biased hiring practices.
- Finance: Credit scoring algorithms can perpetuate economic disparities by favoring established credit profiles, disadvantaging individuals with limited credit history.
The implications of algorithmic bias are far-reaching, eroding trust in AI systems and perpetuating social inequalities. This also raises significant privacy concerns, as individuals from marginalized groups may face disproportionate surveillance and discrimination.
The Shift from Notice-and-Choice to a Proactive Model of Data Protection
Traditionally, privacy protection relied on the concept of “notice-and-choice,” where individuals were informed about data collection practices and given the choice to opt-in or opt-out. However, in the era of AI and algorithmic decision-making, this model is increasingly insufficient.
A proactive model of data protection is emerging, which focuses on the following principles:
- Transparency: AI systems should be transparent about their data sources, algorithms, and decision-making processes. This empowers individuals to understand how their data is used.
- Explainability: AI systems should provide clear explanations for their decisions, particularly in critical domains like healthcare and finance. This allows individuals to challenge decisions and seek recourse when necessary.
- Risk Assessment: Organizations should conduct risk assessments to identify and mitigate potential biases in AI systems before deployment.
- Audits and Accountability: Regular audits and accountability mechanisms should be in place to monitor AI systems’ performance and compliance with fairness and privacy standards.
AI Policy Options for Privacy Protection
Addressing the complex interplay between AI and privacy requires a multifaceted approach that combines technical innovation, regulatory frameworks, and ethical considerations. Here are some policy options that can help protect privacy in an AI-driven world:
Direct Measures Against Discrimination
- Anti-Discrimination Laws: Enact and enforce laws that explicitly prohibit discrimination in AI systems. These laws should cover aspects such as employment, housing, lending, and criminal justice, ensuring that AI algorithms do not perpetuate biases or discriminate against protected groups.
- Fairness Audits: Implement mandatory fairness audits for AI systems used in critical domains. Independent auditors can assess algorithms for bias and discrimination, and organizations must rectify any identified issues.
Accountability Measures
- Transparency Requirements: Mandate transparency in AI systems. Organizations should disclose the use of AI in decision-making processes and provide individuals with access to their data and algorithmic profiles.
- Explainability Standards: Require AI systems to provide clear, understandable explanations for their decisions, particularly in contexts where those decisions have significant consequences for individuals.
- Algorithmic Impact Assessments: Implement requirements for organizations to conduct algorithmic impact assessments to identify and mitigate potential biases and privacy risks.
Ethical and Technical Safeguards
- Ethical AI Frameworks: Develop and promote ethical AI frameworks that prioritize privacy, fairness, and accountability. Encourage organizations to adopt these frameworks voluntarily.
- Privacy by Design: Promote the integration of privacy considerations into the design and development of AI systems. Privacy-by-design principles ensure that privacy is a foundational component of AI projects.
- Data Minimization: Encourage the collection and retention of only necessary data, limiting the exposure of sensitive information.
International Cooperation
- International Standards: Collaborate on the development of international standards for AI ethics and privacy. A unified approach can help address cross-border data flows and global challenges associated with AI.
- Information Sharing: Facilitate the sharing of information and best practices among governments, organizations, and civil society to foster a global understanding of AI’s impact on privacy.
Conclusion
In this exploration of safeguarding data in an AI-driven world, we’ve journeyed through the transformative landscape where technology meets privacy. AI’s rapid ascent has brought forth both remarkable possibilities and pressing challenges. We’ve witnessed the explosive growth of data, delved into the nuances of Big Data, and examined how AI’s integration into our lives impacts privacy. Moreover, we’ve scrutinized the complexities of algorithmic bias, the evolving nature of personal data, and the imperative shift towards proactive data protection.
As we navigate this ever-evolving AI universe, one thing remains clear: the delicate balance between technological advancement and personal privacy is paramount. Our collective responsibility lies in staying informed, championing ethical AI practices, and fostering global cooperation. Only then can we ensure that the AI-driven world we shape is not just technologically advanced but also one where privacy and dignity are upheld, offering a future where both innovation and trust flourish hand in hand.
Leave a Reply